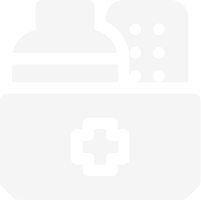
Personalised Medicine
The purpose of this use case is to utilise EVENFLOW technology to forecast events related to critical stages in tumour evolution, or adverse medication side-effects and outcomes in oncological, virtual patient applications. Emphasis will be put on the generation of reliable virtual patient data, which will be integrated in the online neuro-symbolic learning process of EVENFLOW. Initial data generation will be based on deep generative models, such as Variational Autoencoders (VAEs).
As a next step, disentangled latent spaces will be extracted from the learnt VAE models. such spaces map covarying, unobserved (latent) factors in the data generation process (e.g. the gene expression profiles of a cohort of patients) to specific observed parameters (generative factors) of the model that generates the data, (e.g. dysregulation of a set of genes). Crucially, such disentangled latent spaces may be used to infer probabilistic trajectories connecting distinct points in time in the generated data, related to e.g. stages of tumour evolution, or events related to the progression of a patient’s status over the course of a treatment.
Events along such trajectories are related to temporally correlated attributes of the data (generative factors), such as molecular indicators of pharmacologic responses to a therapeutic intervention. The goal will then be to learn complex event patterns from such probabilistic event-based trajectories and use these patterns and the generated data to learn how to forecast the occurrence of complex events from early signs.